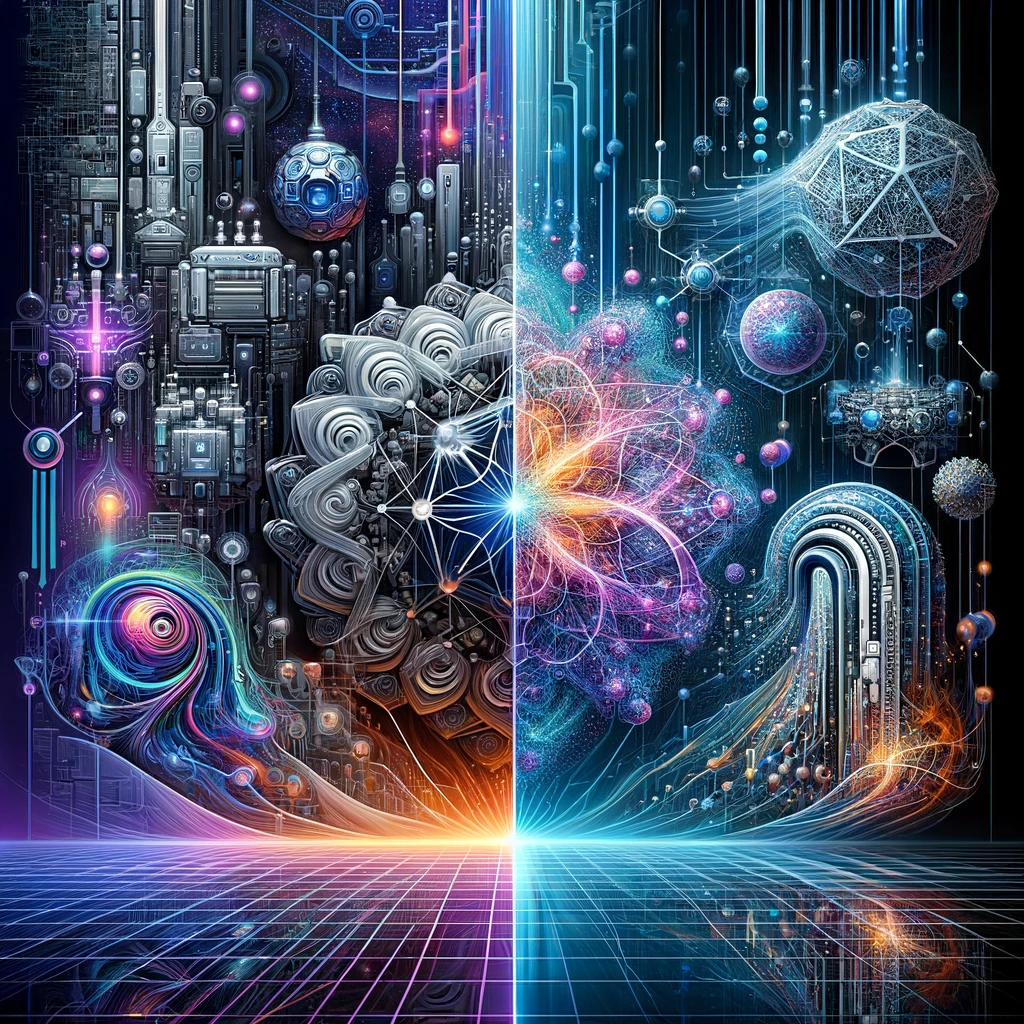
Understanding the Evolution of AI: Transformer Models vs. Traditional Deep Learning - A 2024 Perspective
Posted on January 20, 2024
The realm of artificial intelligence (AI) has seen remarkable advancements, particularly with the rise of transformer models. As we step into 2024, let's explore how these models differ from traditional deep learning models and why these differences matter.
Introduction:
The realm of artificial intelligence (AI) has seen remarkable advancements, particularly with the rise of transformer models. As we step into 2024, let’s explore how these models differ from traditional deep learning models and why these differences matter.
The Architectural Revolution: Transformers vs. CNNs/RNNs
Transformer models represent a significant shift from traditional architectures like CNNs and RNNs. Unlike CNNs, which excel in image processing, and RNNs, which handle sequential data, transformers are designed around a self-attention mechanism. This enables them to process entire sequences of data in parallel, enhancing their ability to understand context and manage complex language tasks.
Visual Comparison of CNN and Transformer Architectures
Scaling Up: The Parameter Game
The scale of transformer models like GPT-3 is staggering when compared to traditional CNNs. While CNNs have parameters in the millions, transformers like GPT-3 boast billions. This vast scale allows them to capture nuances in language generation and translation with unprecedented depth.
Infographic of Parameters Scale
Beyond Text: Expanding Applications
Originally designed for language processing, transformers are now making waves in other domains like image and audio processing. Their ability to handle different types of data makes them versatile tools in the AI toolkit.
Transformers in Various Domains
Emerging Trends: Small Language Models and GenAI
Recent trends point towards the development of Small Language Models (SLMs) and General AI (GenAI). SLMs offer efficiency and can be tailored to specific tasks, providing a more focused approach compared to the ‘one-size-fits-all’ nature of larger models. GenAI represents a broader integration of AI across various industries, promising innovation and productivity leaps.
The Future Landscape: Ethical AI and Human Collaboration
Looking ahead, the focus is shifting towards the ethical use of AI and collaboration between humans and AI systems. The potential for AI to reshape industries is significant, but it must be harnessed responsibly to ensure it enhances human well-being and addresses global challenges.
Conclusion:
As we embrace 2024, the potential of AI and transformer models continues to grow. From enhancing language processing to transforming other domains, these advancements signal a new era in technology. The key lies in leveraging these tools to enrich our lives and solve complex global issues.